Chapter 13. AI, Visitor Experience, and Museum Operations: A Closer Look at the Possible
- Ariana French
- Elena Villaespesa
Artificial Intelligence (AI) technologies bring notable opportunities for museums to learn more about their visitors, play with their collection data and, all combined, influence how people experience a museum. AI and the potential impact of these technologies in enhancing the user experience has had an increasingly important presence in the last few Museum Computer Network conferences. This essay will reflect on the emergence of AI in museums and its role in museum operations as these tools are becoming more widely used. As a result of these reflections, this essay will consider the practical implications of AI and how museums can become informed consumers of these emerging technologies.
The term AI was coined by John McCarthy in 1956 who affirmed that: ‘every aspect of learning or any other feature of intelligence can in principle be so precisely described that a machine can be made to simulate it’.1 This concept has shifted over the last few decades and there have been fluctuations in the application of AI technologies. Nowadays, there is no widely accepted definition of exactly what AI is, and what disciplines are included in the field.
There are multiple classifications of AI technologies2 and among the most common methods we find are computer vision, machine learning, robotics, and natural language processing. All of these methods offer a way to speed processes which would otherwise involve human labor and costs, such as language translation or image identification. Although we may be in another hype phase of the term “AI,” popularity of the tools are normalizing its usage in research and practice. Moreover, while some outputs generated by AI are still very raw and experimental, some applications are becoming an expected functionality by users, such as recommendations, browsing by tags, image recognition from taking a photo or getting a response from a voice assistant.
Museums and AI: The landscape today
Every day, more of our museum visitors’ lives are subtly shaped by AI-driven technologies. Within museum operations, AI has the potential to positively influence how quickly and effectively museums respond to these evolving visitor needs and expectations. Two of the main motivations that drive users to a museum’s website are planning a future visit and accessing the collection to research, learn, or feel inspired.3 We can apply these main website use cases to the types of questions people ask to voice assistants, chatbots, or robots, and how visitors interact with museums, using other interactive interfaces. The usage of voice and image search is rapidly increasing. Are museums ready to respond to these changing information search behaviors?
Voice processing and visual recognition are among the technologies classified under the AI heading. However, there are other technologies which offer new ways of interacting with museums and museum data. A search of AI initiatives in museums on the internet, case studies presented in past museum conferences, and journal databases surfaces sixty-one examples (Figure 1). The broad language used to describe AI initiatives makes searching for use cases a daunting task and the true number of examples is most likely greater than sixty-one. Nonetheless, a clear trend can be identified: the implementation of these technologies has really taken off in the past three years. Examples include chatbots for visitor engagement, predictive analytics for visitor attendance, sentiment analysis for visitor comments, and many more.
Two main areas of data analysis in the museum sector are collection information management and visitor research and evaluation. AI can contribute to the enhancement of the collection data with techniques to clean it up, discover new information, create relationships among data sets, or generate classifications and tags automatically. In the area of visitor research, museums have visitor data from ticket purchases, membership subscriptions, website analytics, Wi-Fi connections, and many other data sources. Applying AI techniques to explore, examine and draw conclusions based on this data can enrich the museum visitor’s experience. Furthermore, museums are required to justify both their private and public funding and also demonstrate the economic and social impact they have on society. AI can potentially be implemented with this aim of demonstrating meaningful insights into the value of the museum experience and its impact on the visitor.
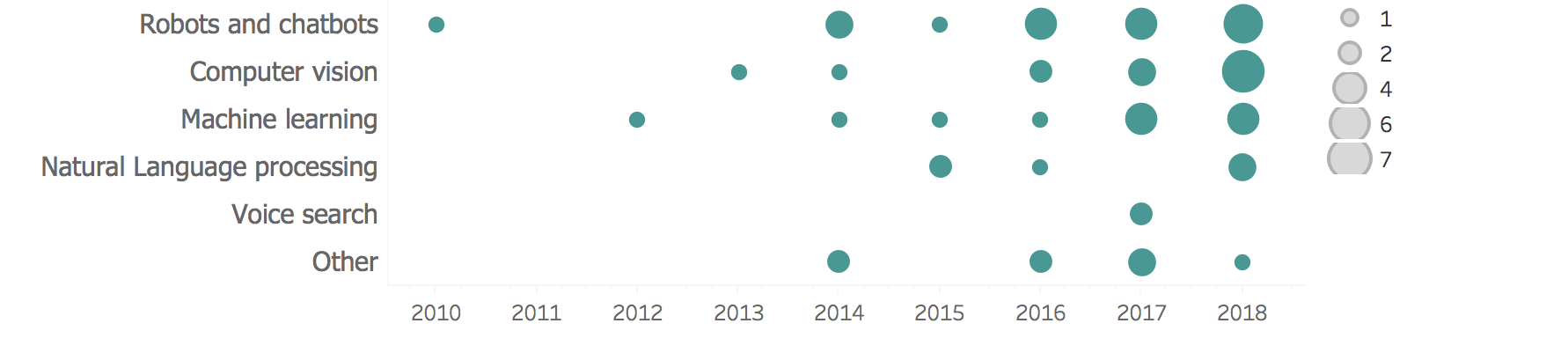
As illustrated in Figure 1, the list of AI technologies is long and, therefore, their potential application in museums is also extensive. We aim to review in this text three key areas where AI is emerging in museums: computer vision for collections information, machine learning for visitor data, and the trending popularity of voice assistants. While the examples listed are far from a full picture of the types of applications for AI, this section aims to illustrate how museums are making use of these tools. Additionally, it aims to provide the necessary context for future reflection about the challenges and limitations of the tools, such as management of these initiatives, ethics, and algorithmic biases.
One of the most promising technologies is the application of computer vision for museum collections. Machines can extract individual elements from the digital object records with a speed that would take humans ages to generate. Running an algorithm on collection data can result in data visualizations of all the object dimensions4 or the identification of faces or landmarks5—offering curatorial staff novel ways of analyzing, researching, and describing museum collections. Insights can be surfaced on new ways of exploring collections by color, for example on the Cooper Hewitt Smithsonian Design Museum6 and Dallas Art Museum websites,7 filters by shape and direction of lines, or space and light as seen on the Barnes Foundation website.8 In another playful example of using AI to generate new ways of collections interaction, the Harvard Art Museum’s public API includes machine-generated data in its “Magic Message,” where users receive image fragments based on a sentence , or “Face Match” that invites the user to add faces to the corresponding bodies.9
While the opportunities for new insights and faster data analysis are tantalizing, AI isn’t a “one size fits all” set of technologies. Many applications are still rough and unproven. For example, a complex task for AI is to detect the elements depicted in a museum object to generate subject tags. This possibility has been tested, but results delivered so far haven’t been completely satisfactory.10 Browsing by subject terms brings a valuable way for users to discover objects, but online collections interfaces can be very limited in their ability to integrate these features. While these applications cause excitement in the museum community, there are clear challenges raised from these projects in terms of accuracy and relevancy which need to be carefully considered.
The examples explored so far illustrate how AI can enhance museum collections information. Looking to visitor data as a source for AI-driven insights, we find a growing interest in the sector to use machine learning for visitation pattern prediction and experience evaluation. The volume of user data generated in museums continues to increase rapidly. Data is collected from the visitors’ physical experience but also from all the different digital touchpoints of that journey: web, social media, ticketing, and mobile apps, among others. The application of AI to analyze and visualize this data brings an opportunity for museums to better understand their audiences and create personalized and engaging experiences.
For AI to be most effective, there has to be a clearly defined problem to solve. When it comes to museums, traditional data analysis includes audience segmentation, visitation predictions, market research to target prospective members, and visitor experience evaluation. AI brings new possibilities in tackling the examination of these existing datasets, and can also facilitate gathering new data for these purposes. For example, visitor experience has traditionally been evaluated through questioning visitors about their satisfaction. With the aid of AI, analyzing posts from social media or ratings from tourism websites can bring new insights into the visitor experience.
Machine learning, in particular, has been applied to find patterns about visitation in order to predict future trends, to analyze visitors’ comments on social media, and identify potential members, among other cases. An example of the usage of external data to discover insights about the visitor experience is the analysis of comments on TripAdvisor. To understand the broad tones of sentiment reflected in tens of thousands of comments, sentiment analysis and topic modeling were applied to generate insights.11 Sentiment analysis of reviews can be considered a valuable data point in understanding visitor sentiment along with other metrics like an organization’s Net Promoter Score, a measure used to gauge satisfaction and loyalty. To bring a more focused understanding of visitor satisfaction, sentiment analysis along with entity analysis can give more detailed insights into how visitors experienced specific aspects of a museum, such as an exhibition.
As in many areas of emerging technologies, pilot projects are essential steps to explore where the opportunities exist for AI and museums. These opportunities are projected to grow exponentially over the next few years.12 However, as is often the case with new technologies, the hype of the possible can obscure the reality of the feasible. For enterprise-level operations, the reality is intimidating: Many machine learning projects involve significant time and resources to prepare data inputs in a “computer-friendly” format. When considering AI-supported solutions in operational contexts, the value proposition continues to be unclear for many museums due to upfront resource investments and subsequent opportunity costs.
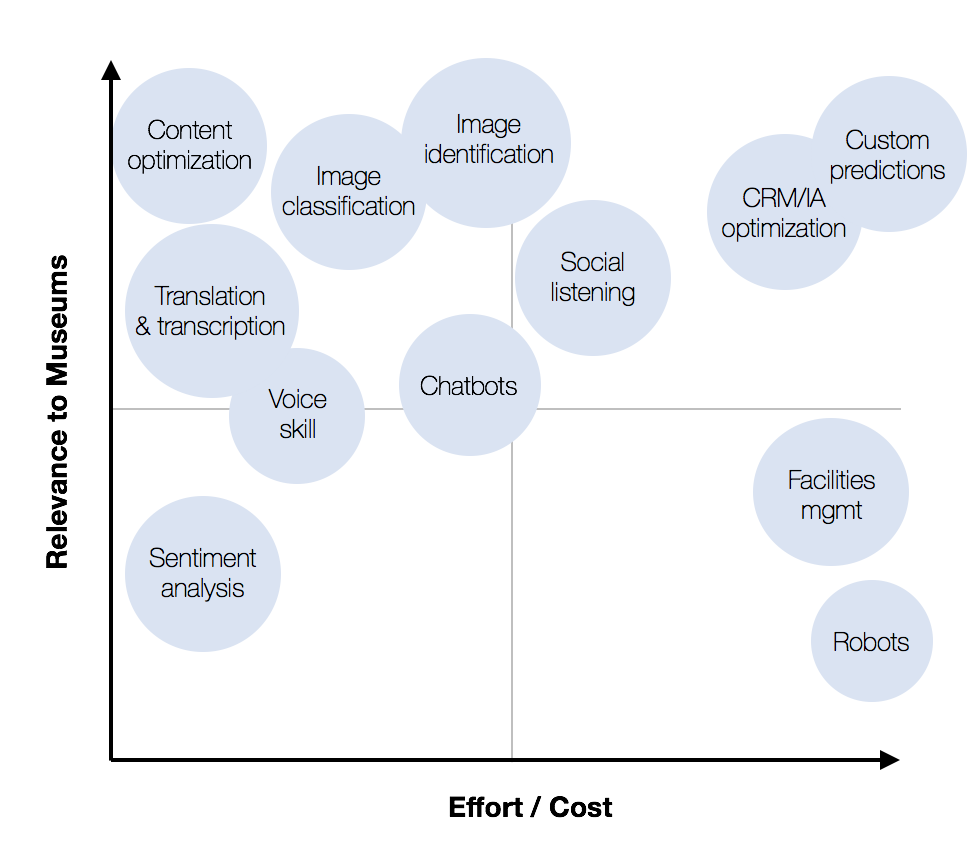
Figure 2 can be interpreted as an attempt to align relevancy to museum operations against investment for popular AI technologies. It can also be read as a “build vs. buy” comparison, where the left quadrants represent what could be applied with the help of a partner or in-house resource, and right quadrants describing what likely involves a commercial product or partner at additional cost. (That said, any of the technologies shown could be increased in complexity, thereby driving up cost or effort.)
In Figure 2, the areas with high relevance to museums and a lower barrier to entry are largely related to museum content. Website text, collection images, tweets, brochures, Instagram posts—and more!—are familiar parts of museum operations. Yet the framing of AI usage in museums is often circumscribed by a “pilot project” approach and isolated from more established digital initiatives.
As barriers to entry continue to drop and use cases become more tangible, opportunities to integrate AI into museum operations may be reconsidered and usher in an era of accelerated change. Today’s denormalized and scattered soup of visitor data sources might be tomorrow’s engine for AI-driven insights! In the meantime, one change that’s well underway is an expansion from touch-centric inputs (like keyboards and touch screens) towards a growing use of AI: voice-assisted technologies.
Apple’s Siri, Google’s Home, and Amazon’s Echo voice assistants (and embedded versions of these technologies in other devices) are among the most popular AI-driven voice assisted technologies. In most of these platforms, query results can be spoken or displayed as text, using algorithms to derive results from web content.
Today, around 20% of search engine queries are voice-assisted, and this number is projected to grow to as much as 50% of all queries in 2020.13 “Searching the web” and other voice-assisted interactions is now happening in more contexts with the spread of voice technologies in “smart” devices. As AI improves its ability to parse and “speak,” the days of touch and sight as primary means of interaction with smart devices and computers may be numbered. Some predict that as AI evolves, more web content will be turned into an on-demand audio experience as it is “spoken” by AI-assisted technologies, creating a new age of content consumption.14
Ensuring digital content is accessible to AI-driven technologies is a challenge—an operational one—that’s already here. But meeting this challenge doesn’t mean starting from scratch; it borrows heavily from best practices in search engine optimization and information architecture design.15 Unique, authoritative text, video, and images with standard markup (for example, the schema.org framework) is good practice for both visual and voice-assisted search. Along with well-structured content, text that’s written in a conversational style supports a more human-like exchange for voice assistant users.16
Nearly half of daily voice-assisted searches involve local organizations and the “featured snippet” in Google’s search results drives up to 80% of what Google returns.17 On Amazon’s Echo devices and similar assistants, voice query results are limited to the top result, and additional prompts are necessary if more information is needed. The interface for screen-based voice assistants (such as Siri on an iPhone) is likewise truncated with limited voice options to follow up an initial query.
With all of this in mind, visitor information on a museum’s website—such as hours, parking information, and accessible entrances—represents a tangible, current optimization opportunity for museums. As the number of AI-assisted platforms, devices, and modalities grow, ensuring that museum visitors receive clear, device-appropriate information in the moment they need it is imperative.
Another imperative is clear, in the dawning age of AI: There’s never been a better time to reevaluate museum-published digital content. Perhaps more than computer vision, AI-driven analyses of visitor sentiment, and other pilot projects noted here, it’s the attention to context and content—how, where, and when museum visitors need information across devices and touchpoints and via different means of consumption (such as voice, keyboard, and screen)—that is a growing and AI-ready opportunity. This is especially true as the museum sector evolves outreach strategies and visitors’ expectations are likewise shaped by algorithms in personalized digital experiences.
AI and ethics
Outside the museum sector, machine learning algorithms are being applied in life-changing ways. From judicial sentencing to medical diagnoses, AI can impact human lives profoundly, and not always for the better.18 AI is a complex set of algorithms created by humans, tested by humans, and trained by humans—and, not surprisingly—retains human biases. Data scientist Cathy O’Neil, author of Weapons of Math Destruction, called algorithms “opinions embedded in code.”19 The subjectivity of these algorithms and the inherently complex, “black box” characteristics of AI have led to a precarious reality: AI-generated results that neither humans nor machines can explain.20 As use cases for AI grow, an urgent need for a human-centered approach—including literacy on AI, understanding bias in AI, and all of the ethical implications—grows with it.
As noted earlier in this essay, machine learning algorithms can speed the time it takes to surface insights within collections and museum audiences. The marketing appeal of AI to bring about more nimble operations isn’t new to customer relationship management (CRM) software vendors; Blackbaud and Salesforce offer platforms with “AI” components to help museums reach new audiences in record time. But any application of AI in development initiatives should be considered carefully, given known problems of bias and questionable outcomes. Especially where constituent data is concerned, an informed approach to what AI can (and should) provide can help museums ensure that problems are defined sharply, expectations for AI-supported contributions are set appropriately, and resources are invested wisely.
As we face an increasingly technology-enabled future, exactly how AI will change daily museum activities and public life remains to be seen. Museums have long played a role in matters of social justice and occupy a singular position in mediating the cultural dialogue of AI, its impact on societies, and ethics.21 Continued pilot projects around the applications of AI, dialogue within (and outside) the sector, attention to opportunities in museum operations (such as optimizing content for voice-assisted technologies), and understanding topics like algorithmic bias will help advance this discourse as a fundamentally human one.
Conclusion: Artificial intelligence and its place in museums
Any time you talk about an emerging technology, museums have an important role to play teaching the public about it. Artificial intelligence is going to be incredibly important in shaping the world we live in, in profound ways. We need to understand the technology and the issues it raises.”
—Elizabeth Merritt, director of the American Alliance of Museums’ Center for the Future of Museums.22
If the breathless media coverage and accelerating policy talk are any indication, AI will shape lives for years to come. From breakthroughs in medicine to better email spam filters, the applications for AI and machine learning grow every year. In the museum sector, AI offers a range of opportunities, from new modes of engaging with collections to institutional advancement initiatives and beyond. However, barriers to entry can be high before sustainable, operational impact can be proven with many applications of AI; upfront investments to “build from scratch” are out of reach for many museums. In spite of this reality (and in contrast to the hype), there is at least one accessible, present-day use case for AI in museum operations: Well-developed digital content is a foundation for engaging new audiences through voice-assisted technologies.
Looking ahead, museums are uniquely positioned to foster dialogues on matters of AI, ethics, impact, and opportunity. If the popularity of AI-related conference topics continues its upward trend and public usage of these technologies likewise grows, we may soon be able to reflect upon the question of museum readiness beyond a “pilot project” approach to AI. Questioning, evaluating, and continuing to push the dialogue on these technologies is a recent but increasingly relevant topic for museum conferences and similar forums, particularly those with a human-centered vision. Without a global authority to regulate the evolution of AI, it’s up to an educated public to discern its ethical (and practical) applications. As AI evolves ever-more humanized modes of interaction—through touch, voice, sight, and sound—the museum community can offer an educational and safe space through which to explore the complex world of artificial intelligence.
Notes
- John McCarthy et al., “A Proposal for the Dartmouth Summer Research Project on Artificial Intelligence, August 31, 1955,” AI Magazine 27, no. 4 (December 15, 2006): 12–12, https://doi.org/10.1609/AIMAG.V27I4.1904. ↩
- “Artificial Intelligence: How Knowledge Is Created, Transference, and Used,” Report. Elsevier. 2018, https://iatranshumanisme.com/wp-content/uploads/2018/12/ACAD_RL_RE_AI-Report_WEB.pdf (accessed February 10, 2019); Franceso Corea, “AI Knowledge Map: How To Classify AI Technologies,” Forbes, August 2018, https://www.forbes.com/sites/cognitiveworld/2018/08/22/ai-knowledge-map-how-to-classify-ai-technologies/#c605fa47773f (accessed February 10, 2019); Peter Stone et al., “Artificial Intelligence and Life in 2030: One Hundred Year Study on Artificial Intelligence,” Stanford University, September 2016, https://ai100.stanford.edu/2016-report (accessed February 10, 2019). ↩
- Elena Villaespesa and John Stack, “Finding the Motivation behind a Click: Definition and Implementation of a Website Audience Segmentation,” in MW2015: Museums and the Web 2015, 2015, https://mw2015.museumsandtheweb.com/paper/finding-the-motivation-behind-a-click-definition-and-implementation-of-a-website-audience-segmentation/ (accessed February 10, 2019); Silvia Filippini Fantoni, Rob Stein, and Gray Bowman, “Exploring the Relationship between Visitor Motivation and Engagement in Online Museum Audiences,” in MW12: Museums and the Web 2012, 2012, https://www.museumsandtheweb.com/mw2012/papers/exploring_the_relationship_between_visitor_mot (accessed February 10, 2019). ↩
- James Davenport, “The Dimensions of Art,” If We Assume, November 2013, https://www.ifweassume.com/2013/11/the-dimensions-of-art.html (accessed February 10, 2019). ↩
- Sara Robinson, “When Art Meets Big Data: Analyzing 200,000 Items from The Met Collection in BigQuery,” Google Cloud Blog, August 2017, https://cloud.google.com/blog/products/gcp/when-art-meets-big-data-analyzing-200000-items-from-the-met-collection-in-bigquery (accessed February 10, 2019). ↩
- Cooper-Hewitt Smithsonian Design Museum, “The Collection,” accessed March 1, 2019, https://collection.cooperhewitt.org/ (accessed February 10, 2019). ↩
- Dallas Museum of Art, “DMA Collection Online,” accessed March 1, 2019, https://collections.dma.org/ (accessed February 10, 2019). ↩
- Barnes Foundation, “Barnes Collection Online,” accessed March 1, 2019, https://collection.barnesfoundation.org/ (accessed February 10, 2019). ↩
- Harvard Art Museums, “IIIF Explorer,” accessed March 1, 2019, http://iiif-explorer.herokuapp.com/ (accessed February 10, 2019). ↩
- Adrian Hindle, “Automated Image Analysis with IIIF. Using Artificial Intelligence for Bulk Image Analysis,” Cogapp Medium, June 2017, https://blog.cogapp.com/automated-image-analysis-with-iiif-6594ff5b2b32 (accessed February 10, 2019); Shelley Bernstein, “Using Computer Vision to Tag the Collection,” Barnes Foundation Medium, October 2017, https://medium.com/barnes-foundation/using-computer-vision-to-tag-the-collection-f467c4541034 (accessed February 10, 2019). ↩
- Ariana French, “On Artificial Intelligence, Museums, and Feelings,” 2018, https://medium.com/@CuriousThirst/on-artificial-intelligence-museums-and-feelings-598b7ba8beb6 (accessed February 10, 2019); Victoria D. Alexander, Grant Blank, and Scott A. Hale, “TripAdvisor Reviews of London Museums: A New Approach to Understanding Visitors,” Museum International 70, no. 1–2 (January 11, 2018): 154–65, https://doi.org/10.1111/muse.12200 (accessed February 10, 2019). ↩
- Bennat Berger, “AI-Enabled Technologies Could Help Museums Survive the Digital Age,” VentureBeat, November 2017, https://venturebeat.com/2017/11/06/ai-enabled-technologies-could-help-museums-survive-the-digital-age/ (accessed February 10, 2019). ↩
- Jayson DeMers, “Why You Need To Prepare For A Voice Search Revolution,” Forbes, January 2018, https://www.forbes.com/sites/jaysondemers/2018/01/09/why-you-need-to-prepare-for-a-voice-search-revolution/#3322221134af (accessed February 10, 2019). ↩
- Rebecca Sentance, “The Future of Voice Search: 2020 and Beyond,” Econsultancy, July 2018, https://econsultancy.com/the-future-of-voice-search-2020-and-beyond/ (accessed February 10, 2019). ↩
- Therese Sullivan, “Appreciating How Metadata Makes AI Possible,” Building Context, January 2017, https://buildingcontext.me/2017/01/12/appreciating-how-metadata-makes-ai-possible/ (accessed February 10, 2019). ↩
- Roger Montti, “Google Answers How to Optimize Content for Voice Search,” Search Engine Journal, January 2018, https://www.searchenginejournal.com/voice-search-optimization/231319/ (accessed February 10, 2019). ↩
- Christi Olson, “Voice Search Isn’t the next Big Disrupter, Conversational AI Is,” MarTech Today, October 2018, https://martechtoday.com/voice-search-isnt-the-next-big-disrupter-conversational-ai-is-226537 (accessed February 10, 2019); Saima Salim, “Voice-Assisted Search: The Future of Internet Queries (Infographic),” Digital Information World, October 2018, https://www.digitalinformationworld.com/2018/10/infographic-the-state-of-mobile-voice-search.html (accessed February 10, 2019). ↩
- Jason Tashea, “Courts Are Using AI to Sentence Criminals. That Must Stop Now,” WIRED, April 2017, https://www.wired.com/2017/04/courts-using-ai-sentence-criminals-must-stop-now/ (accessed February 10, 2019); Jeremy Hsu, “Can a Crowdsourced AI Medical Diagnosis App Outperform Your Doctor?,” Scientific American, August 2017, https://www.scientificamerican.com/article/can-a-crowdsourced-ai-medical-diagnosis-app-outperform-your-doctor/ (accessed February 10, 2019); Julia Angwin et al., “Machine Bias,” ProPublica, May 2016, https://www.propublica.org/article/machine-bias-risk-assessments-in-criminal-sentencing (accessed February 10, 2019). ↩
- Yves Smith, “Data Scientist Cathy O’Neil: ‘Algorithms Are Opinions Embedded in Code’,” Naked Capitalism, August 2017, https://www.nakedcapitalism.com/2017/08/data-scientist-cathy-oneil-algorithms-opinions-embedded-code.html (accessed February 10, 2019). ↩
- Cliff Kuang, “Can A.I. Be Taught to Explain Itself?,” The New York Times, November 2017, https://www.nytimes.com/2017/11/21/magazine/can-ai-be-taught-to-explain-itself.html (accessed February 10, 2019). ↩
- Kate McLeod, “The Role Museums Play in Social Activism,” Americans for the Arts, 2017, https://www.americansforthearts.org/2017/08/02/the-role-museums-play-in-social-activism (accessed February 10, 2019). ↩
- Jane L. Levere, “Artificial Intelligence, Like a Robot, Enhances Museum Experiences,” The New York Times, October 2018, https://www.nytimes.com/2018/10/25/arts/artificial-intelligence-museums.html (accessed February 10, 2019). ↩
Bibliography
- Alexander, Victoria D., Grant Blank, and Scott A. Hale. “TripAdvisor Reviews of London Museums: A New Approach to Understanding Visitors.” Museum International 70, no. 1–2 (January 11, 2018): 154–65. https://doi.org/10.1111/muse.12200.
- Angwin, Julia, Jeff Larson, Surya Mattu, and Lauren Kirchner. “Machine Bias.” ProPublica, May 2016. https://www.propublica.org/article/machine-bias-risk-assessments-in-criminal-sentencing (accessed February 10, 2019).
- “Artificial Intelligence: How Knowledge Is Created, Transference, and Used,” Report. Elsevier. 2018. https://iatranshumanisme.com/wp-content/uploads/2018/12/ACAD_RL_RE_AI-Report_WEB.pdf (accessed February 10, 2019).
- Barnes Foundation. “Barnes Collection Online.” Accessed March 1, 2019. https://collection.barnesfoundation.org/ (accessed February 10, 2019).
- Berger, Bennat. “AI-Enabled Technologies Could Help Museums Survive the Digital Age.” VentureBeat, November 2017. https://venturebeat.com/2017/11/06/ai-enabled-technologies-could-help-museums-survive-the-digital-age/ (accessed February 10, 2019).
- Bernstein, Shelley. “Using Computer Vision to Tag the Collection.” Barnes Foundation Medium, October 2017. https://medium.com/barnes-foundation/using-computer-vision-to-tag-the-collection-f467c4541034 (accessed February 10, 2019).
- Cooper-Hewitt Smithsonian Design Museum. “The Collection | Collection of Cooper Hewitt, Smithsonian Design Museum.” https://collection.cooperhewitt.org/ (accessed February 10, 2019).
- Corea, Franceso. “AI Knowledge Map: How To Classify AI Technologies.” Forbes, August 2018. https://www.forbes.com/sites/cognitiveworld/2018/08/22/ai-knowledge-map-how-to-classify-ai-technologies/#c605fa47773f (accessed February 10, 2019).
- Dallas Museum of Art. “DMA Collection Online.” https://collections.dma.org/ (accessed February 10, 2019).
- Davenport, James. “The Dimensions of Art.” If We Assume, November 2013. https://www.ifweassume.com/2013/11/the-dimensions-of-art.html (accessed February 10, 2019).
- DeMers, Jayson. “Why You Need To Prepare For A Voice Search Revolution.” Forbes, January 2018. https://www.forbes.com/sites/jaysondemers/2018/01/09/why-you-need-to-prepare-for-a-voice-search-revolution/#3322221134af (accessed February 10, 2019).
- Filippini Fantoni, Silvia, Rob Stein, and Gray Bowman. “Exploring the Relationship between Visitor Motivation and Engagement in Online Museum Audiences.” In MW12: Museums and the Web 2012, 2012. https://www.museumsandtheweb.com/mw2012/papers/exploring_the_relationship_between_visitor_mot (accessed February 10, 2019).
- French, Ariana. “On Artificial Intelligence, Museums, and Feelings,” 2018. https://medium.com/@CuriousThirst/on-artificial-intelligence-museums-and-feelings-598b7ba8beb6 (accessed February 10, 2019).
- ———. “On Artificial Intelligence, Museums, and Spaghetti,” 2018. https://medium.com/@CuriousThirst/on-artificial-intelligence-museums-and-spaghetti-b107cf1b4dc9 (accessed February 10, 2019).
- Harvard Art Museums. “IIIF Explorer | Harvard Art Museums.” http://iiif-explorer.herokuapp.com/ (accessed February 10, 2019).
- Hindle, Adrian. “Automated Image Analysis with IIIF. Using Artificial Intelligence for Bulk Image Analysis.” Cogapp Medium , June 2017. https://blog.cogapp.com/automated-image-analysis-with-iiif-6594ff5b2b32 (accessed February 10, 2019).
- Hsu, Jeremy. “Can a Crowdsourced AI Medical Diagnosis App Outperform Your Doctor?” Scientific American, August 2017. https://www.scientificamerican.com/article/can-a-crowdsourced-ai-medical-diagnosis-app-outperform-your-doctor/ (accessed February 10, 2019).
- Kuang, Cliff. “Can A.I. Be Taught to Explain Itself?” The New York Times, November 2017. https://www.nytimes.com/2017/11/21/magazine/can-ai-be-taught-to-explain-itself.html (accessed February 10, 2019).
- Levere, Jane L. “Artificial Intelligence, Like a Robot, Enhances Museum Experiences.” The New York Times, October 2018. https://www.nytimes.com/2018/10/25/arts/artificial-intelligence-museums.html (accessed February 10, 2019).
- McCarthy, John, Marvin L. Minsky, and Claude E. Shannon. “A Proposal for the Dartmouth Summer Research Project on Artificial Intelligence - August 31, 1955.” AI MAGAZINE, December 15, 2006. https://doi.org/10.1609/AIMAG.V27I4.1904 (accessed February 10, 2019).
- McLeod, Kate. “The Role Museums Play in Social Activism.” Americans for the Arts, 2017. https://www.americansforthearts.org/2017/08/02/the-role-museums-play-in-social-activism (accessed February 10, 2019).
- Montti, Roger. “Google Answers How to Optimize Content for Voice Search.” Search Engine Journal, January 2018. https://www.searchenginejournal.com/voice-search-optimization/231319/ (accessed February 10, 2019) (accessed February 10, 2019).
- Olson, Christi. “Voice Search Isn’t the next Big Disrupter, Conversational AI Is.” MarTech Today, October 2018. https://martechtoday.com/voice-search-isnt-the-next-big-disrupter-conversational-ai-is-226537 (accessed February 10, 2019).
- Robinson, Sara. “When Art Meets Big Data: Analyzing 200,000 Items from The Met Collection in BigQuery.” Google Cloud Blog, August 2017. https://cloud.google.com/blog/products/gcp/when-art-meets-big-data-analyzing-200000-items-from-the-met-collection-in-bigquery (accessed February 10, 2019).
- Salim, Saima. “Voice-Assisted Search: The Future of Internet Queries (Infographic).” Digital Information World, October 2018. https://www.digitalinformationworld.com/2018/10/infographic-the-state-of-mobile-voice-search.html (accessed February 10, 2019).
- Sentance, Rebecca. “The Future of Voice Search: 2020 and Beyond.” Econsultancy, July 2018. https://econsultancy.com/the-future-of-voice-search-2020-and-beyond/ (accessed February 10, 2019).
- Smith, Yves. “Data Scientist Cathy O’Neil: ‘Algorithms Are Opinions Embedded in Code’;” Naked Capitalism, August 2017. https://www.nakedcapitalism.com/2017/08/data-scientist-cathy-oneil-algorithms-opinions-embedded-code.html (accessed February 10, 2019).
- Stone, Peter, Rodney Brooks, Erik Brynjolfsson, Ryan Calo, Oren Etzioni, Greg Hager, Julia Hirschberg, et al. “Artificial Intelligence and Life in 2030: One Hundred Year Study on Artificial Intelligence.” Stanford University. Retrieved July 2 (2016). https://ai100.stanford.edu/2016-report (accessed February 10, 2019).
- Sullivan, Therese. “Appreciating How Metadata Makes AI Possible.” Building Context, January 2017. https://buildingcontext.me/2017/01/12/appreciating-how-metadata-makes-ai-possible/ (accessed February 10, 2019).
- Tashea, Jason. “Courts Are Using AI to Sentence Criminals. That Must Stop Now.” WIRED, April 2017. https://www.wired.com/2017/04/courts-using-ai-sentence-criminals-must-stop-now/ (accessed February 10, 2019).
- Villaespesa, Elena, and John Stack. “Finding the Motivation behind a Click: Definition and Implementation of a Website Audience Segmentation.” In MW2015: Museums and the Web 2015, 2015. https://mw2015.museumsandtheweb.com/paper/finding-the-motivation-behind-a-click-definition-and-implementation-of-a-website-audience-segmentation/ (accessed February 10, 2019).